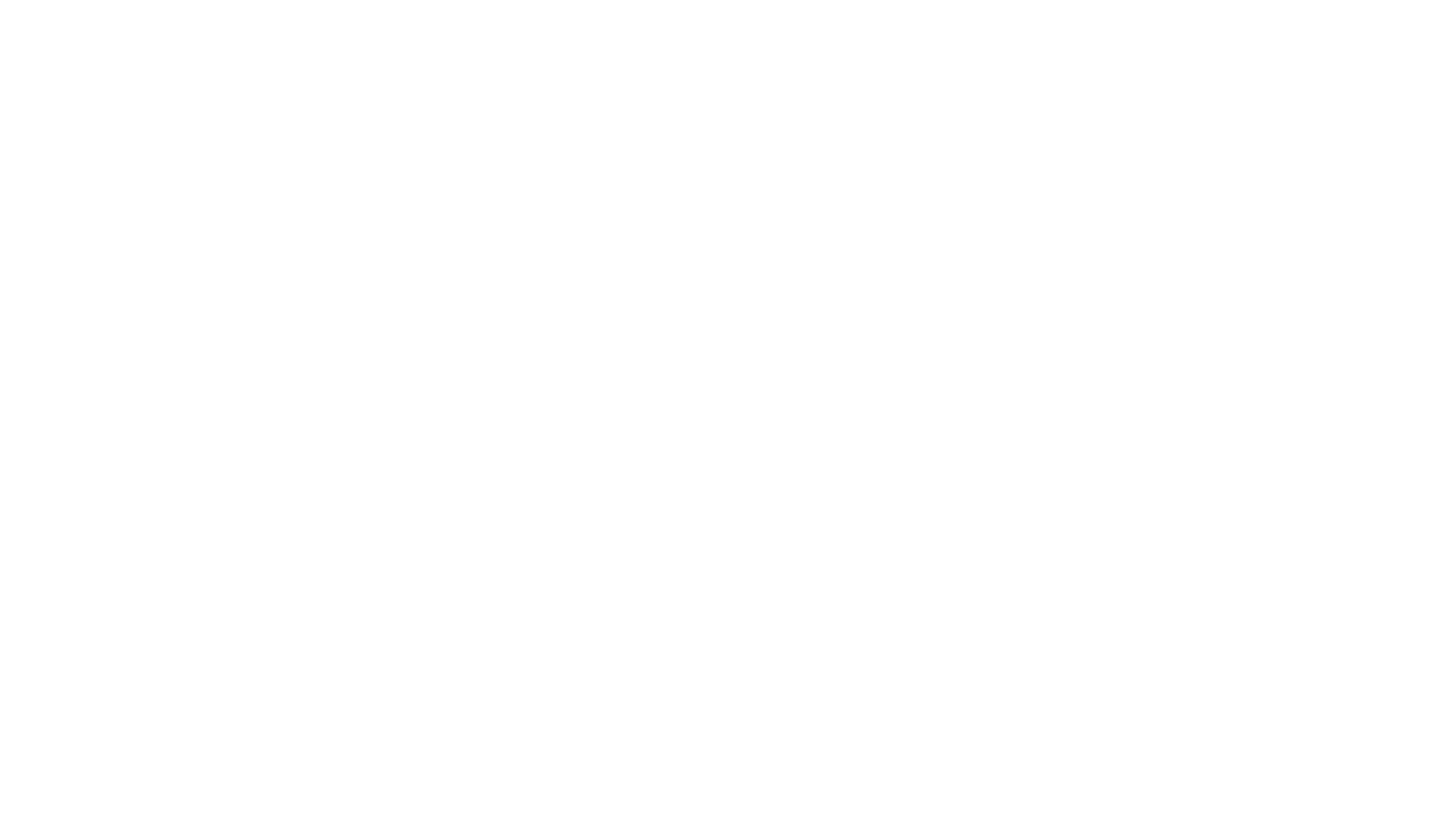
How much can machine learning boost wind returns?
Unpredictability is one of wind energy’s biggest limitations. Power grids are designed to work with large controllable electricity generators and at present have little storage capacity.
Unpredictability is one of wind energy’s biggest limitations. Power grids are designed to work with large controllable electricity generators and at present have little storage capacity.
By contrast, the wind isn’t controllable and it’s hard to know how it’ll blow. But companies have been improving. Wind companies and researchers have worked on potential solutions in the past decade. We are now seeing a new wave of artificial intelligence and machine learning projects.
For example, Vestas and its subsidiary Utopus Insight secured funding this week from the Australian Renewable Energy Agency to apply deep learning techniques to develop short-term wind energy forecasting solutions in the country.
Swedish utility Vattenfall has acquired Dutch start-up Senfal, which offers software that uses machine learning algorithms to trade wind and solar power automatically on short-term energy markets.
But it is an initiative by tech giant Google that has particularly caught our attention.
London-based Google subsidiary DeepMind revealed last month it has been testing an algorithm for the past year that is able to predict wind energy output. DeepMind is an artificial intelligence company founded in 2010, which was acquired by Google in 2014 for £400m.
The company has been working on a machine learning algorithm that it has applied to a Google wind portfolio totalling 700MW in the US. Using weather forecasts and historical turbine data, the algorithm has been able to predict wind turbines’ output 36 hours ahead of time.
It isn’t hard to see why this could be highly valuable. According to DeepMind, this could boost the value of the wind energy in the portfolio by 20% in the past year compared to the status quo.
In simple terms, the algorithm would let wind companies make hourly commitments to the power grid a day in advance, and this would make wind energy more valuable for wind farm owners because they can sell it when prices are more favourable. The researchers say this is the first time machine learning has been used to predict the output of a larger fleet, but it has been used on single turbines before.
In addition, statistical prediction models have been used to forecast wind patterns as well as wind output for shorter amounts of time. The big difference with these models and others is that DeepMind’s algorithm is able to analyse historical weather and wind turbine performance data, and actively learn from them for future predictions.
However, as we saw earlier, Google isn’t alone in its quest of making artificial intelligence and wind energy work together. In fact, wind farm developers and owners have been taking note of the value that machine learning solutions can add to their portfolios.
For example, US developer Invenergy teamed up last year with software maker NarrativeWave, which develops algorithms that crunch years of operating data. They are working on making turbines more reliable by predicting seasonal wind patterns, propeller performance and the likelihood of tech failures.
And Chicago-based software producer Uptake Technologies is working with Berkshire Hathaway and Pattern Energy to boost the output of existing turbines and improving their efficiency by using artificial intelligence software configured for energy and performance predictions.
This shows wind companies are increasingly aware that machine learning could really help boost revenue of their portfolios. Google may now look to sell its technology to wind developers, but other companies have been taking steps too to develop in-house solutions based on artificial intelligence.
The use of machine learning has already spread across several industries, including banking, fintech and healthcare. Now it is wind’s turn.